Understanding how Privacy works in B2B AI products
A comprehensive analysis on the different levels of Privacy available for AI adoption
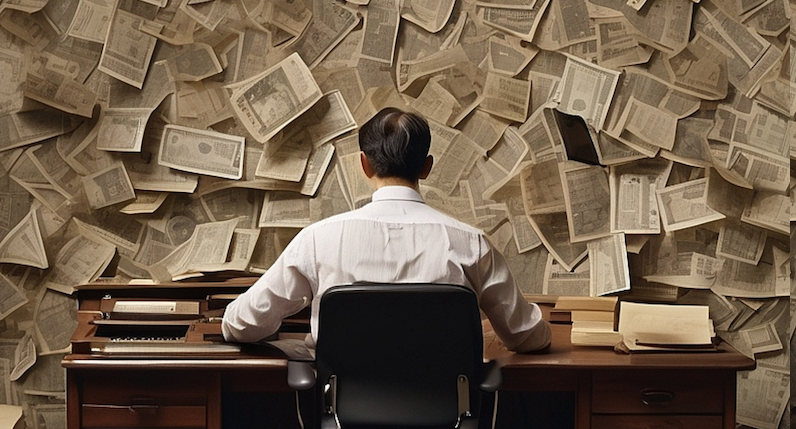
Data privacy is a major blocker for AI adoption. To make it even worse, the current landscape of B2B AI products makes it hard for companies to understand the different alternatives and how their data is really managed, because every solution claims to be “private”.
This article brings some clarity by providing an analysis on the different AI adoption alternatives, ranging from the least private to the most.
Compliant SaaS
Many AI SaaS providers are SOC2, GDPR and even HIPAA certified. However, these certifications do not guarantee data privacy since they are relatively easy to obtain and sometimes are more focused on the internal processes of the companies itself, than ensuring a proper data management.
Moreover, most SaaS make use of third party providers for different sub-processes (LLMs, embeddings, reranking…), resulting in private data being transferred and stored on numerous servers across the Internet, hence losing control over who could tentatively access it if a data breach happens.
Data Anonymization
Privacy-aware SaaS services like Legalfly or Mostly.ai anonymize sensitive data before sending it to the AI layer in charge of running the embeddings, inferences, etc. This approach combines the ease of adoption and power of SaaS with data privacy.
However, it comes with a single point of failure, which is the anonymization algorithm itself: if the anonymization process fails, your data could be exposed. But even if it is 100% anonymous, then the risk is reducing quality of the results due to lack of proper context for the LLM.
Local Execution
AI that runs on your laptop or smartphone, such as PrivateGPT or Proton Scribe, ensures 100% data privacy since no data leaves your device and you can even run everything without Internet connection.
However, your device must be quite powerful (GPU, RAM…), otherwise you will be constrained regarding AI capabilities of the system or even its quality. Furthermore, by executing it in isolation its adoption and collaboration in professional environments is limited or even not feasible.
In-House Development
Implementing an end-to-end, in-house AI solution that runs within the company's infrastructure ensures privacy and does not limit AI capabilities assuming you have the resources to run costly AI services.
However, it requires a significant investment of time, money, and focus, making it suitable only for large enterprises having a dedicated tech team capable of developing everything or those with enough economic muscle to hire these services to consultancy agencies or third parties.
Zylon AI Workspace
At Zylon, we have chosen to provide in-house-level data privacy without the pain of in-house development. We've created a self-contained, ready-to-use AI workspace that is simple to install on-premises, from data centers to all major private clouds.
This means that whatever is the level of data compliance at a company, Zylon inherits it due to the fact of being running within its existing infrastructure. In other words, you can think about Zylon as another piece of your company inner software stack.
Thanks to this, data anonymization is not necessary at all, thus simplifying the AI system and making it perform faster and cheaper because no intermediate steps to anonymize and un-anonymize data are required.
Last but not least, the level of isolation can be completely customized at deploying time: some companies want to run Zylon in their own private clouds (AWS, Azure or GCP); while others want to deploy it in their air-gapped data centers (with or without Internet connection).
We hope this guide and the summary table below helps you navigate the growing offering of AI services.
